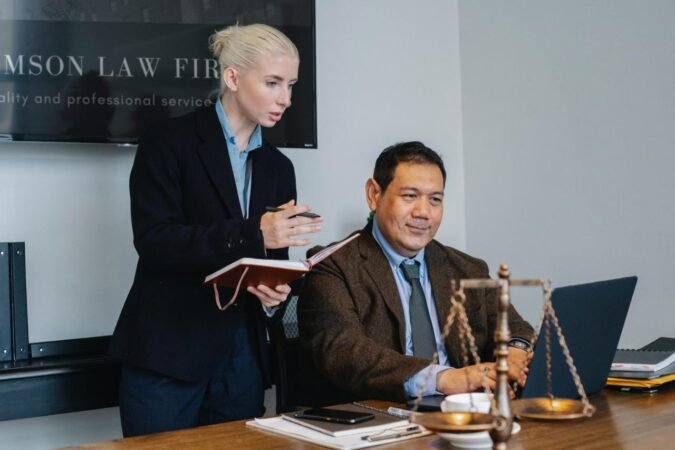
- AI Bias in Legal Decisions: A Comprehensive Guide
-
FAQ about AI Bias in Legal Decisions
- What is AI bias in legal decisions?
- What are the main sources of AI bias?
- How can AI bias impact legal decisions?
- What are the challenges in identifying and addressing AI bias?
- What are potential solutions to reduce AI bias?
- What role do AI developers have in preventing AI bias?
- What role do regulators have in addressing AI bias?
- What can legal professionals do to mitigate AI bias?
- What is the future of AI bias in legal decisions?
AI Bias in Legal Decisions: A Comprehensive Guide
Introduction
Hey there, readers! Are you curious about the role of AI in the legal system? In recent years, there’s been a growing concern about AI bias in legal decisions. This article will dive into the complexities of this topic, exploring its various facets and implications. So, grab a virtual cup of coffee and let’s delve into the world of AI and legal justice!
AI has the potential to revolutionize many industries, including the legal field. However, as with any technology, it comes with its own set of challenges. One of the biggest concerns is the potential for bias to creep into AI systems used in legal decision-making.
Types of AI Bias in Legal Decisions
Algorithmic Bias
AI algorithms are trained on vast amounts of data. If the data used to train the algorithm is biased, the algorithm itself will be biased. This can lead to unfair or inaccurate decisions in legal cases. For example, if an algorithm used to predict recidivism is trained on data that is biased against certain racial groups, it may overpredict the likelihood of those individuals committing future crimes.
Data Bias
Bias can also be introduced into AI systems through the data that is collected. For example, if a dataset used to train an AI system includes more data from one particular group of people than another, the system may be biased towards that group. This can lead to unfair or inaccurate decisions in legal cases.
Human Bias
Bias can also be introduced into AI systems through the humans who design and implement them. For example, if the developers of an AI system have biases against certain groups of people, those biases may be reflected in the system’s design or implementation. This can lead to unfair or inaccurate decisions in legal cases.
Implications of AI Bias in Legal Decisions
The potential implications of AI bias in legal decisions are vast and far-reaching. These include:
Unfair Sentencing
AI algorithms are increasingly used to make sentencing decisions in criminal cases. If these algorithms are biased, they could lead to unfair or excessive sentences for certain groups of people.
Discrimination in Hiring and Promotion
AI algorithms are also used to make hiring and promotion decisions. If these algorithms are biased, they could lead to discrimination against certain groups of people in the workplace.
Denial of Benefits
AI algorithms are also used to make decisions about who is eligible for government benefits. If these algorithms are biased, they could lead to the denial of benefits to certain groups of people who are rightfully entitled to them.
Case Studies of AI Bias in Legal Decisions
There have been several high-profile cases of AI bias in legal decisions in recent years. These include:
COMPAS Algorithm
The COMPAS algorithm is a risk assessment tool used to predict recidivism. However, a study by ProPublica found that the algorithm was biased against black defendants, overpredicting their likelihood of committing future crimes.
Northpointe Algorithm
The Northpointe algorithm is another risk assessment tool used to predict recidivism. However, a study by the University of California, Berkeley found that the algorithm was biased against black and Hispanic defendants, overpredicting their likelihood of committing future crimes.
Amazon AI Hiring Tool
In 2018, Amazon scrapped an AI hiring tool after it was found to be biased against women. The tool was designed to identify promising job candidates, but it was found to be less likely to recommend women for high-paying jobs.
Table: Examples of AI Bias in Legal Decisions
Case | Type of Bias | Impact |
---|---|---|
COMPAS Algorithm | Algorithmic bias | Overprediction of recidivism for black defendants |
Northpointe Algorithm | Algorithmic bias | Overprediction of recidivism for black and Hispanic defendants |
Amazon AI Hiring Tool | Human bias | Discrimination against women in hiring |
Conclusion
AI bias in legal decisions is a serious problem that has the potential to undermine the fairness and impartiality of the legal system. It is important to be aware of the risks of AI bias and to take steps to mitigate them. This includes using unbiased data to train AI algorithms, testing algorithms for bias, and auditing AI systems for fairness.
If you’re interested in learning more about AI bias, here are some additional resources:
FAQ about AI Bias in Legal Decisions
What is AI bias in legal decisions?
Answer: AI bias refers to the tendency of AI algorithms to make unfair or inaccurate predictions based on historical data that contains biases. In legal settings, this can lead to discriminatory outcomes, such as unfair sentencing or biased jury selection.
What are the main sources of AI bias?
Answer: AI bias can arise from:
- Data bias: Data used to train AI algorithms may contain biases that reflect existing social inequalities.
- Algorithmic bias: The algorithms themselves may contain biases that favor certain groups over others.
- Human bias: Humans involved in the development and deployment of AI can introduce biases based on their own preconceptions.
How can AI bias impact legal decisions?
Answer: AI bias can lead to:
- Unfair sentencing: AI algorithms may predict recidivism or risk of future crime based on biased data, leading to harsher sentences for certain groups.
- Biased jury selection: AI can be used to select jurors based on demographics or other factors that perpetuate biases against certain groups.
- Discriminatory bail decisions: AI algorithms may predict flight risk or dangerousness based on biased data, leading to unfair bail decisions.
What are the challenges in identifying and addressing AI bias?
Answer: Challenges include:
- Lack of transparency: AI algorithms are often complex and opaque, making it difficult to identify and understand sources of bias.
- Data limitations: Addressing bias requires access to diverse and unbiased data, which can be challenging to obtain.
- Ethical considerations: Addressing bias often involves trade-offs between fairness and efficiency.
What are potential solutions to reduce AI bias?
Answer: Solutions include:
- Data audits: Regularly auditing data used for AI algorithms to identify and mitigate biases.
- Algorithmic fairness: Developing algorithms that explicitly consider and reduce bias.
- Human oversight: Involving humans in the decision-making process to identify and correct biased predictions.
What role do AI developers have in preventing AI bias?
Answer: AI developers have a responsibility to:
- Awareness: Understand the potential for bias and take steps to mitigate it.
- Transparency: Provide documentation and explanations of AI algorithms to promote transparency.
- Collaboration: Work with legal experts, ethicists, and other stakeholders to develop ethical and unbiased AI systems.
What role do regulators have in addressing AI bias?
Answer: Regulators have a role to:
- Set standards: Develop guidelines and regulations for AI development and deployment that address bias concerns.
- Monitor compliance: Enforce regulations and hold AI developers accountable for biased systems.
- Promote transparency: Encourage AI developers to provide accessible information about their algorithms and decision-making processes.
What can legal professionals do to mitigate AI bias?
Answer: Legal professionals can:
- Educate themselves: Understand the potential for AI bias and its implications for legal decision-making.
- Scrutinize AI systems: Critically examine AI algorithms for bias and challenge biased predictions.
- Advocate for transparency: Demand transparency in AI development and deployment to promote accountability.
What is the future of AI bias in legal decisions?
Answer: The future of AI bias in legal decisions depends on ongoing efforts to identify and address biases. Collaboration between AI developers, regulators, legal professionals, and researchers will be crucial in developing ethical and unbiased AI systems for the justice system.