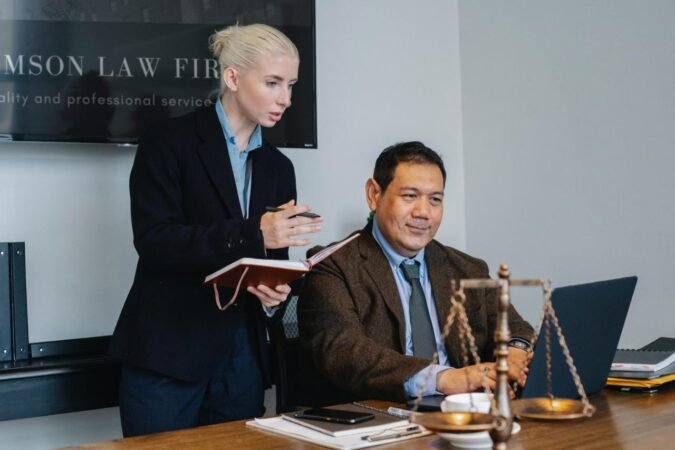
- Introduction: Hi there, readers!
- Deep Learning: A Primer
- Enhancing Legal Research
- A Detailed Table of Applications
- Conclusion: Delve into Deep Learning for Legal Success
-
FAQ about Deep Learning in Legal Research
- 1. What is deep learning?
- 2. How is deep learning being used in legal research?
- 3. What are the benefits of using deep learning in legal research?
- 4. What are the challenges of using deep learning in legal research?
- 5. What is the future of deep learning in legal research?
- 6. How can I learn more about deep learning in legal research?
- 7. What are some examples of deep learning applications in legal research?
- 8. What are the ethical implications of using deep learning in legal research?
- 9. How can I ensure that deep learning models are used ethically in legal research?
- 10. What is the future of deep learning in legal research?
Introduction: Hi there, readers!
Welcome to our comprehensive guide on the captivating intersection of deep learning and legal research. As legal professionals and enthusiasts, we’re thrilled to delve into this cutting-edge technology and explore its transformative impact on the justice system.
In this article, we’ll unravel the mysteries of deep learning, its applications in legal research, and its potential to revolutionize the way legal professionals approach their work. So, sit back, relax, and let’s embark on this enlightening journey together!
Deep Learning: A Primer
What is Deep Learning?
Deep learning is a subset of machine learning that employs artificial neural networks (ANNs) with multiple hidden layers. These layers enable ANNs to learn complex patterns and relationships in data, making them adept at tasks such as image recognition, natural language processing, and predictive modeling.
Deep Learning in Legal Research
The legal domain is replete with vast amounts of unstructured text, making it an ideal candidate for deep learning applications. By leveraging deep learning models, legal professionals can automate tasks such as:
- Document Classification: Classifying legal documents into categories, such as contracts, court orders, or case summaries.
- Legal Text Summarization: Generating concise summaries of lengthy legal documents, saving time and effort.
- Case Law Prediction: Predicting the outcome of legal cases based on historical data and patterns.
Enhancing Legal Research
Legal Document Analysis
Deep learning models can perform in-depth analysis of legal documents, extracting key concepts, entities, and relationships. This enables lawyers to quickly grasp the essence of complex contracts, regulations, or court decisions.
Predictive Legal Analytics
Predictive legal analytics harnesses deep learning models to forecast legal outcomes and identify potential risks. By analyzing historical case data, these models can provide valuable insights and enhance strategic decision-making for attorneys.
Legal Discovery and E-Discovery
Deep learning accelerates the legal discovery and e-discovery process by automating document review and identification. Models can sift through massive datasets, searching for relevant information based on predefined criteria, reducing time and expenses.
A Detailed Table of Applications
Application | Description |
---|---|
Document Classification | Categorizing legal documents into various classes, such as contracts, pleadings, or witness statements |
Legal Text Summarization | Generating concise and informative summaries of lengthy legal documents |
Case Law Prediction | Predicting the likely outcome of legal cases based on historical data and patterns |
Legal Due Diligence | Identifying potential legal risks and liabilities associated with business transactions |
Legal Research Assistance | Assisting legal professionals in finding relevant case law, statutes, and other legal materials |
Legal Chatbots | Providing instant access to legal information and guidance through automated chatbots |
Legal Decision-Making Support | Enhancing legal decision-making by providing data-driven insights and predictive modeling |
Conclusion: Delve into Deep Learning for Legal Success
Readers, we hope this article has ignited your curiosity and inspired you to explore the transformative potential of deep learning in legal research. By embracing this technology, legal professionals can optimize their workflows, enhance their decision-making, and ultimately deliver exceptional legal services.
For further exploration, we invite you to check out our other articles on cutting-edge technologies in the legal field. Remember, the journey to legal innovation begins with a single step. Take that step today and unlock the power of deep learning for justice!
FAQ about Deep Learning in Legal Research
1. What is deep learning?
Deep learning is a type of machine learning that uses artificial neural networks to learn from data. These networks are made up of layers of interconnected nodes, each of which learns to recognize a specific pattern in the data.
2. How is deep learning being used in legal research?
Deep learning is being used in a variety of ways to assist legal researchers, including:
- Automated document review: Deep learning models can be trained to review documents and identify relevant information, such as key facts, legal issues, and citations.
- Predictive analytics: Deep learning models can be used to predict the outcome of legal cases based on historical data.
- Legal research: Deep learning models can be used to search for legal precedents and other relevant materials, and to summarize the findings.
3. What are the benefits of using deep learning in legal research?
- Increased efficiency: Deep learning models can automate many of the tasks that are currently performed manually by legal researchers, saving time and money.
- Improved accuracy: Deep learning models can be trained to identify patterns in data that humans may miss, leading to more accurate results.
- New insights: Deep learning models can help legal researchers to identify new patterns and relationships in the data, leading to new insights into the law.
4. What are the challenges of using deep learning in legal research?
- Data availability: Deep learning models require large amounts of data to train, and legal data can be difficult to obtain.
- Bias: Deep learning models can be biased, reflecting the biases in the data they are trained on. This can lead to inaccurate or unfair results.
- Explainability: Deep learning models can be difficult to explain, making it difficult to understand how they arrive at their conclusions.
5. What is the future of deep learning in legal research?
Deep learning is a rapidly developing field, and it is likely to have a significant impact on legal research in the years to come. As deep learning models become more sophisticated and more data becomes available, they will be able to perform more complex tasks and provide more valuable insights to legal researchers.
6. How can I learn more about deep learning in legal research?
There are a number of resources available to learn more about deep learning in legal research, including:
- Books and articles: There are a number of books and articles that have been published on the topic of deep learning in legal research.
- Online courses: There are a number of online courses available that teach the basics of deep learning and its applications to legal research.
- Conferences and workshops: There are a number of conferences and workshops on deep learning in legal research that are held throughout the year.
7. What are some examples of deep learning applications in legal research?
Some examples of deep learning applications in legal research include:
- Automated document review: Deep learning models can be used to review documents and identify relevant information, such as key facts, legal issues, and citations. This can save legal researchers a significant amount of time and effort.
- Predictive analytics: Deep learning models can be used to predict the outcome of legal cases based on historical data. This can help legal researchers to make more informed decisions about their cases.
- Legal research: Deep learning models can be used to search for legal precedents and other relevant materials, and to summarize the findings. This can help legal researchers to find the information they need more quickly and easily.
8. What are the ethical implications of using deep learning in legal research?
There are a number of ethical implications to consider when using deep learning in legal research, including:
- Bias: Deep learning models can be biased, reflecting the biases in the data they are trained on. This can lead to inaccurate or unfair results.
- Explainability: Deep learning models can be difficult to explain, making it difficult to understand how they arrive at their conclusions. This can make it difficult to trust the results of deep learning models.
- Privacy: Deep learning models can be used to analyze large amounts of data, including personal data. This raises concerns about privacy and data protection.
9. How can I ensure that deep learning models are used ethically in legal research?
There are a number of steps that can be taken to ensure that deep learning models are used ethically in legal research, including:
- Collecting unbiased data: It is important to collect unbiased data to train deep learning models. This can be done by using a variety of data sources and by taking steps to remove bias from the data.
- Making models explainable: It is important to make deep learning models explainable so that users can understand how they arrive at their conclusions. This can be done by using techniques such as interpretable machine learning.
- Protecting privacy: It is important to protect privacy when using deep learning models to analyze data. This can be done by using anonymized data and by taking steps to protect the data from unauthorized access.
10. What is the future of deep learning in legal research?
Deep learning is a rapidly developing field, and it is likely to have a significant impact on legal research in the years to come. As deep learning models become more sophisticated and more data becomes available, they will be able to perform more complex tasks and provide more valuable insights to legal researchers.