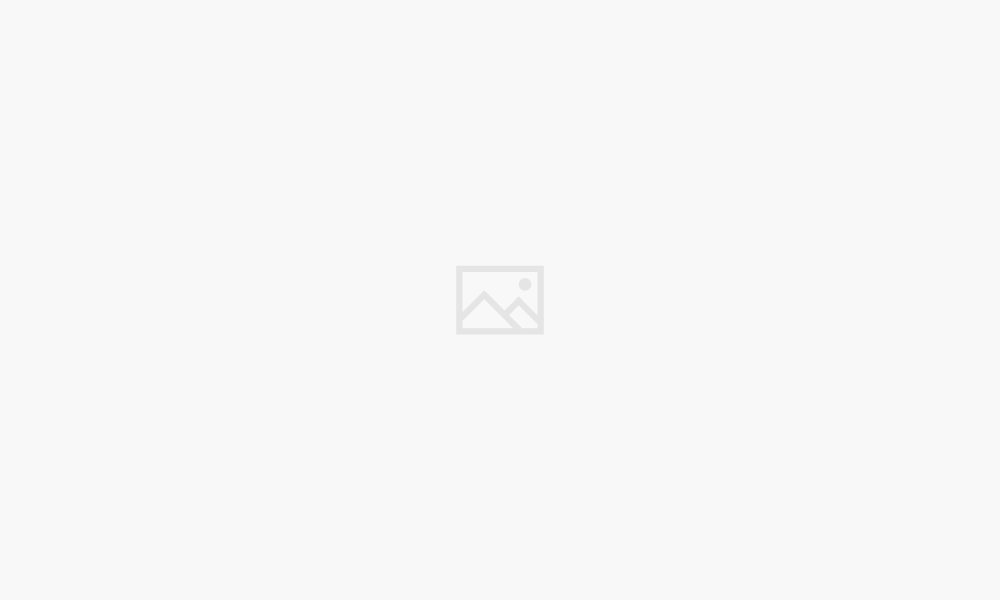
- Electricity Generation Forecasting: A Comprehensive Guide
- Conclusion
-
FAQs about Electricity Generation Forecasting
- What is electricity generation forecasting?
- Why is electricity generation forecasting important?
- How are electricity generation forecasts made?
- What factors affect electricity generation forecasts?
- How far in advance can electricity generation be forecasted?
- What are the challenges of electricity generation forecasting?
- How can forecasting accuracy be improved?
- What are the benefits of accurate electricity generation forecasting?
- What are the emerging trends in electricity generation forecasting?
Electricity Generation Forecasting: A Comprehensive Guide
Greetings readers,
Today, we embark on a journey into the fascinating realm of electricity generation forecasting. In an era marked by the increasing complexities of the energy landscape, accurate and reliable electricity generation forecasts hold immense value for grid operators, utilities, and other stakeholders alike. Join us as we delve into the intricacies of forecasting, exploring the methods, applications, and challenges associated with this critical aspect of power system management.
Section 1: The Importance of Electricity Generation Forecasting
1.1 The Need for Accurate Predictions
Electricity generation forecasting plays a pivotal role in ensuring the stability and efficiency of power systems. Accurate forecasts enable grid operators to anticipate future electricity demand, optimize generation resources, and minimize the risk of outages. By precisely predicting generation output, utilities can efficiently schedule maintenance activities, avoid costly over- or under-generation, and contribute to overall grid reliability.
1.2 Applications in Market Operations
Electricity generation forecasting also has significant applications in market operations. Energy traders rely on forecasts to assess market conditions, optimize bidding strategies, and manage risk. Furthermore, forecasts provide valuable insights for renewable energy integration, helping to balance intermittent sources like solar and wind with dispatchable generation.
Section 2: Methods and Techniques
2.1 Statistical Time Series Analysis
Statistical time series analysis is a commonly employed method for electricity generation forecasting. It involves analyzing historical data to identify patterns and trends. Time series models, such as autoregressive integrated moving average (ARIMA), aim to capture the underlying dynamics of the time series and make predictions based on past observations.
2.2 Numerical Weather Prediction
Numerical weather prediction (NWP) models are another valuable tool for forecasting electricity generation from renewable sources like solar and wind. These models simulate atmospheric conditions to predict weather patterns, which can then be used to estimate the availability of renewable energy resources. The accuracy of NWP models has improved significantly in recent years, making them increasingly reliable for forecasting purposes.
Section 3: Challenges and Uncertainties
3.1 Intermittent Renewable Energy Sources
The integration of intermittent renewable energy sources, such as solar and wind, poses significant challenges for electricity generation forecasting. The stochastic nature of these sources makes it difficult to predict their output accurately, particularly in the short term. To address this challenge, probabilistic forecasting methods are being developed to quantify the uncertainty associated with renewable energy generation.
3.2 Changes in Energy Consumption Patterns
Changing energy consumption patterns, influenced by factors such as technology adoption, economic growth, and population demographics, can also impact the accuracy of electricity generation forecasts. Forecasters must continually monitor these trends and adjust their models accordingly to maintain high levels of precision.
Section 4: Forecast Data and Performance Evaluation
Table: Electricity Generation Forecasting Data and Metrics
Data Source | Metric | Description |
---|---|---|
Historical Generation Data | Root Mean Square Error (RMSE) | Measures the average error between actual and forecasted generation |
Weather Forecasts | Mean Absolute Percentage Error (MAPE) | Calculates the average percentage error in forecasted generation |
Market Prices | Correlation Coefficient (R) | Assesses the relationship between forecasted and actual prices |
4.1 Forecast Evaluation Criteria
The accuracy of electricity generation forecasts is typically evaluated using various statistical metrics, including RMSE, MAPE, and R. These metrics measure the discrepancy between actual and forecasted values and provide a quantitative assessment of forecast performance.
Section 5: Future Outlook and Emerging Trends
The future of electricity generation forecasting holds exciting prospects, with advancements in machine learning and artificial intelligence (AI) poised to enhance forecast accuracy further. AI-powered models can leverage vast datasets and complex algorithms to capture intricate relationships and improve prediction capabilities. Additionally, the increasing adoption of smart grids and the Internet of Things (IoT) is generating real-time data that can be utilized to refine forecasts and respond more effectively to dynamic grid conditions.
Conclusion
Electricity generation forecasting is a critical aspect of power system operations, providing valuable insights to grid operators, utilities, and market participants alike. By embracing advanced forecasting methods and addressing the challenges associated with intermittent renewable energy sources and changing consumption patterns, we can empower the energy industry with the knowledge it needs to navigate the complexities of the future and deliver reliable, resilient, and sustainable electricity to homes and businesses worldwide.
We invite you to explore our other articles for further insights into the fascinating world of energy forecasting and its applications in grid management, renewable energy integration, and market operations.
FAQs about Electricity Generation Forecasting
What is electricity generation forecasting?
Electricity generation forecasting is the process of estimating the amount of electricity that will be generated by a power plant or system over a specific period.
Why is electricity generation forecasting important?
Accurate forecasting is essential to ensure a reliable and efficient electricity supply. It helps utilities avoid over- or under-producing electricity, minimize costs, and plan for future investments.
How are electricity generation forecasts made?
Various methods are used, including:
- Statistical models: Analyze historical data to identify patterns and trends.
- Numerical weather prediction models: Predict weather conditions that affect renewable energy generation (e.g., wind, solar).
- Machine learning algorithms: Identify complex relationships in data to improve forecast accuracy.
What factors affect electricity generation forecasts?
- Weather conditions (e.g., temperature, wind speed)
- Load demand
- Fuel prices
- Power plant outages
How far in advance can electricity generation be forecasted?
Forecasts can range from short-term (hours or days) to long-term (months or years). The accuracy of the forecast depends on the time horizon.
What are the challenges of electricity generation forecasting?
- Intermittent nature of renewable energy sources
- Uncertainty in weather conditions
- Sudden changes in demand
How can forecasting accuracy be improved?
- Incorporating new data sources (e.g., smart meters, sensors)
- Utilizing advanced modeling techniques
- Collaborative forecasting between different entities
What are the benefits of accurate electricity generation forecasting?
- Reduces operating costs
- Improves grid reliability
- Facilitates market participation
- Supports decision-making for renewable energy investments
What are the emerging trends in electricity generation forecasting?
- Use of artificial intelligence and machine learning
- Integration of distributed energy resources (e.g., rooftop solar)
- Probabilistic forecasting methods to account for uncertainty