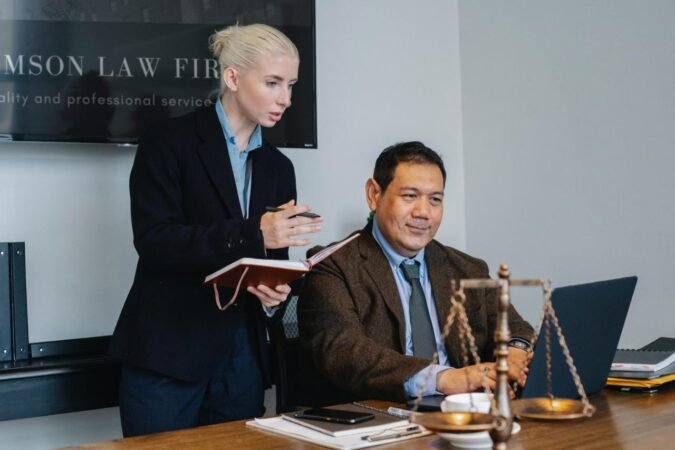
- Greetings, Readers!
- Discriminatory Algorithms: The Pitfall of Bias
- Data Privacy: Navigating the Ethical Labyrinth
- Liability and Accountability: Ascribing Responsibility
- Mitigation Strategies: Navigating the Legal Minefield
- Conclusion: Beyond the Threshold of Risk
-
FAQ about Legal Risks in Machine Learning Models
- 1. What are the potential legal risks associated with using machine learning models?
- 2. How can I mitigate the legal risks of using machine learning models?
- 3. What are the legal implications of using machine learning models for risk assessment?
- 4. How can I use machine learning models without violating privacy laws?
- 5. What are the intellectual property considerations when using machine learning models?
- 6. Who is liable for damages caused by machine learning models?
- 7. How can I ensure compliance with ethical guidelines when using machine learning models?
- 8. What are the legal implications of using machine learning models in autonomous systems?
- 9. How can I stay updated on legal developments related to machine learning models?
- 10. What are the potential legal implications of using machine learning models in the healthcare industry?
Greetings, Readers!
Welcome, dear readers, to the uncharted realm of machine learning models, where algorithms dance and data sings. However, beneath this alluring facade lie potential legal pitfalls that can ensnare the unwary. Let’s embark on a jurisprudential odyssey, navigating the legal risks that accompany these enigmatic entities.
Discriminatory Algorithms: The Pitfall of Bias
Bias Unmasked: The Achilles’ Heel of Machine Learning
Machine learning models, trained on vast troves of data, can inherit biases that mirror societal prejudices. Unchecked, these biases can perpetuate discrimination, leading to unfair outcomes for marginalized individuals. For instance, a hiring algorithm trained on historical data may favor white male applicants, perpetuating a cycle of exclusion.
Mitigating Bias: A Balancing Act
To safeguard against algorithmic bias, organizations must implement rigorous auditing and validation processes. Data scrubbing techniques can help identify and remove biased data points, while algorithmic fairness metrics ensure equitable treatment of all individuals. Additionally, human oversight and review can provide a crucial filter for mitigating potential discriminatory outcomes.
Data Privacy: Navigating the Ethical Labyrinth
Data Collection: A Double-Edged Sword
Machine learning models rely heavily on vast datasets, raising concerns about data privacy. Collecting and processing personal information without consent can violate privacy laws and erosion public trust. Organizations must establish clear data collection policies, adhering to principles of transparency, informed consent, and data minimization.
Data Security: Fortressing the Virtual Realm
The vast amount of data processed by machine learning models presents a tempting target for cybercriminals. Security breaches can compromise sensitive information, leading to identity theft, fraud, and reputational damage. Robust cybersecurity measures, including encryption, intrusion detection systems, and regular vulnerability assessments, are essential for protecting this valuable asset.
Liability and Accountability: Ascribing Responsibility
The Attribution Enigma: Who’s at the Helm?
Machine learning models operate with a degree of autonomy, raising questions about liability and accountability. When a model makes a decision with negative consequences, who is responsible: the creator of the model, the user, or both? Clear legal frameworks are needed to establish liability, ensuring that responsible parties are held accountable for their actions.
Establishing Liability: A Path Forward
Determining liability in cases involving machine learning requires a careful balancing of factors such as the model’s level of autonomy, the foreseeability of harm, and the degree of human oversight. Legal frameworks should provide clear guidelines for apportioning liability, incentivizing responsible development and deployment of machine learning models.
Mitigation Strategies: Navigating the Legal Minefield
Element | Mitigation Strategy
——- | ——–
Discriminatory Algorithms | Implement bias-mitigation measures, such as data scrubbing, algorithmic fairness metrics, and human review.
Data Privacy | Establish clear data collection policies, obtain informed consent, and implement robust cybersecurity measures.
Liability and Accountability | Clarify legal frameworks for establishing liability, ensuring accountability for responsible parties.
Conclusion: Beyond the Threshold of Risk
Dear readers, the legal risks associated with machine learning models are a complex and evolving landscape. By understanding these risks and implementing effective mitigation strategies, organizations can harness the transformative power of machine learning while safeguarding their legal interests. Remember to explore our other articles for further insights into the legal intricacies of artificial intelligence and machine learning.
FAQ about Legal Risks in Machine Learning Models
1. What are the potential legal risks associated with using machine learning models?
- Answer: Machine learning models can pose legal risks, such as discrimination, privacy violations, and intellectual property infringement.
2. How can I mitigate the legal risks of using machine learning models?
- Answer: To mitigate risks, bias in training data should be audited, user consent should be obtained before collecting sensitive data, and intellectual property rights should be respected.
3. What are the legal implications of using machine learning models for risk assessment?
- Answer: Machine learning models may amplify existing biases, leading to unfair discrimination. Transparency and fairness in algorithms should be ensured.
4. How can I use machine learning models without violating privacy laws?
- Answer: Use privacy-enhancing technologies, such as anonymization and differential privacy, to protect sensitive data while leveraging machine learning.
5. What are the intellectual property considerations when using machine learning models?
- Answer: Models often rely on open-source or third-party algorithms. Proper licensing and attribution are crucial to avoid copyright infringement.
6. Who is liable for damages caused by machine learning models?
- Answer: Liability depends on factors such as the model’s purpose, the level of human involvement, and the jurisdiction. Determine liability in contracts and implement risk management strategies.
7. How can I ensure compliance with ethical guidelines when using machine learning models?
- Answer: Adhere to ethical principles, such as fairness, transparency, and accountability. Seek guidance from ethical guidelines and regulatory frameworks.
8. What are the legal implications of using machine learning models in autonomous systems?
- Answer: Autonomous systems pose unique legal challenges related to liability, safety, and ethical considerations. Develop clear policies and regulations for their use.
9. How can I stay updated on legal developments related to machine learning models?
- Answer: Monitor legal news, consult with legal experts, and attend industry conferences to stay informed about evolving regulations and case law.
10. What are the potential legal implications of using machine learning models in the healthcare industry?
- Answer: Machine learning in healthcare raises concerns about data privacy, patient safety, and algorithmic biases. Ensure compliance with HIPAA and other relevant regulations.