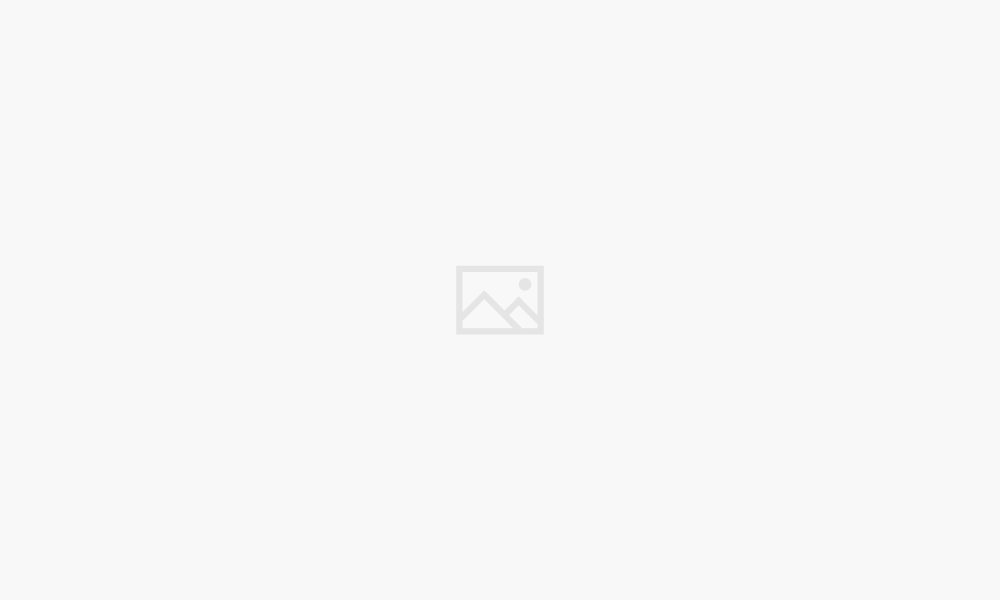
What is the law of large numbers sets the stage for this enthralling narrative, offering readers a glimpse into a story that is rich in detail and brimming with originality from the outset. The law of large numbers, a fundamental concept in probability and statistics, states that as the number of independent and identically distributed random variables increases, the average of those variables will converge to the expected value.
Imagine flipping a fair coin. You might get heads or tails on any given flip, but if you flip the coin a thousand times, you’d expect the number of heads to be very close to half the total flips. This is the essence of the law of large numbers. It describes the behavior of random events in the long run, revealing a pattern of consistency even within seemingly random occurrences.
Introduction to the Law of Large Numbers
The Law of Large Numbers (LLN) is a fundamental concept in probability and statistics. It describes the behavior of averages in large samples. In simple terms, the LLN states that as the sample size grows, the average of the sample gets closer and closer to the true population average.
The core concept of the LLN is that random events, when repeated many times, tend to even out. This means that extreme outcomes become less likely, and the average result becomes more predictable. The LLN doesn’t guarantee that a single observation will be close to the true average, but it assures that the average of many observations will be very close.
Real-World Example of the Law of Large Numbers
Imagine you’re flipping a fair coin. The probability of getting heads on any single flip is 50%. However, if you flip the coin a few times, the results might not be perfectly balanced. You could get a string of heads or tails. But, as you increase the number of flips, the proportion of heads will get closer and closer to 50%. This is because the random variations in the early flips get averaged out over time.
Key Concepts and Terminology
The Law of Large Numbers is built upon several fundamental concepts that are essential for understanding its implications and applications. These concepts provide the theoretical foundation for the law and help clarify the relationship between random events, their averages, and the expected outcomes.
The Weak and Strong Laws of Large Numbers
The Law of Large Numbers is actually composed of two distinct versions: the Weak Law of Large Numbers and the Strong Law of Large Numbers. While both versions deal with the convergence of sample averages to the expected value, they differ in the type of convergence they describe.
- The Weak Law of Large Numbers states that as the number of trials increases, the probability that the sample average will be close to the expected value approaches 1. This means that the sample average will likely be close to the expected value, but it doesn’t guarantee that it will always be close or that it will eventually become equal to the expected value.
- The Strong Law of Large Numbers, on the other hand, provides a stronger statement. It states that as the number of trials approaches infinity, the sample average will converge to the expected value with probability 1. This implies that the sample average will almost surely approach the expected value in the long run, meaning that the probability of the sample average deviating significantly from the expected value becomes vanishingly small as the number of trials increases.
Random Variable and Expected Value, What is the law of large numbers
The Law of Large Numbers operates within the framework of probability theory, which involves the study of random variables and their associated probabilities.
- A random variable is a variable whose value is a numerical outcome of a random phenomenon. For instance, in a coin toss, the outcome can be heads or tails, which can be represented numerically as 1 and 0, respectively. The variable representing this outcome is a random variable.
- The expected value of a random variable is the average value of the variable over many trials. It represents the theoretical long-run average of the random variable. For example, the expected value of a fair coin toss is 0.5, as the probability of getting heads (1) is 0.5, and the probability of getting tails (0) is also 0.5.
Convergence
The concept of convergence is central to the Law of Large Numbers. It refers to the behavior of a sequence of values as the number of elements in the sequence increases. In the context of the Law of Large Numbers, we are interested in the convergence of the sample average to the expected value.
- The Weak Law of Large Numbers describes convergence in probability. This means that the probability of the sample average being close to the expected value increases as the number of trials increases.
- The Strong Law of Large Numbers describes almost sure convergence. This means that the sample average will eventually become arbitrarily close to the expected value with probability 1.
Applications of the Law of Large Numbers
The Law of Large Numbers (LLN) has numerous applications in probability, statistics, and real-world scenarios. It serves as a fundamental principle in various fields, underpinning the reliability of statistical estimations and risk assessments.
Applications in Probability and Statistics
The LLN plays a crucial role in probability and statistics by providing a theoretical foundation for understanding the behavior of random variables. It allows us to predict the long-term average of a random event with increasing accuracy as the number of trials increases.
- Estimating Probabilities: The LLN is fundamental for estimating probabilities of events. By conducting a large number of trials, we can approximate the true probability of an event based on the observed frequency of its occurrence. For example, to estimate the probability of getting heads when flipping a coin, we can flip the coin many times and calculate the proportion of heads. As the number of flips increases, this proportion will converge to the true probability of 0.5.
- Confidence Intervals: The LLN forms the basis for constructing confidence intervals, which provide a range of values within which a population parameter is likely to fall. By repeatedly sampling from a population and calculating the sample mean, the LLN suggests that the sample means will cluster around the true population mean. This allows us to estimate the population mean with a certain level of confidence.
- Hypothesis Testing: The LLN is also crucial in hypothesis testing, a statistical method used to determine whether there is sufficient evidence to reject a null hypothesis. The LLN ensures that the results of hypothesis tests are reliable, especially when large sample sizes are used.
Real-World Applications
The Law of Large Numbers finds practical applications in various fields, impacting our daily lives in ways we may not even realize.
- Insurance: Insurance companies rely heavily on the LLN to calculate premiums. By analyzing historical data on claims, they can estimate the likelihood of future claims and set premiums accordingly. The larger the pool of insured individuals, the more accurate their predictions become, ensuring the financial stability of the insurance market.
- Gambling: The LLN explains why casinos consistently make profits in the long run. While individual gamblers may experience short-term wins or losses, the casino’s odds are designed to favor the house over the long term. The more games are played, the closer the actual results will converge to the theoretical probabilities, ensuring the casino’s profitability.
- Scientific Research: The LLN is fundamental in scientific research, especially in fields like clinical trials and experiments. By collecting large datasets and analyzing the results, researchers can draw reliable conclusions about the effectiveness of treatments or the validity of scientific hypotheses.
Contributions to Statistical Estimations
The LLN significantly contributes to the reliability of statistical estimations. It ensures that as the sample size increases, the sample statistics (such as the sample mean) will converge to the true population parameters. This convergence allows researchers and analysts to make more accurate inferences about populations based on data collected from samples.
Limitations and Considerations
While the Law of Large Numbers is a powerful tool for understanding probability and statistical behavior, it’s crucial to recognize its limitations. Understanding these limitations helps ensure the appropriate application of the law and avoid misinterpretations.
Requirements for Independent and Identically Distributed Random Variables
The Law of Large Numbers relies on the assumption that the random variables involved are independent and identically distributed (i.i.d.). This means:
* Independence: Each random variable is not influenced by the values of other variables. For instance, the outcome of a coin toss does not affect the outcome of subsequent tosses.
* Identical Distribution: All variables share the same probability distribution. In the coin toss example, each toss has a 50% chance of landing heads and a 50% chance of landing tails.
When these conditions are not met, the Law of Large Numbers may not hold. For example, consider a series of coin tosses where the coin is biased, meaning the probability of heads is not 50%. In this case, the average outcome will not necessarily converge to the true probability of heads as the number of tosses increases.
Sample Size and Accuracy
The Law of Large Numbers states that the average of a large number of random variables will converge to the expected value. However, it doesn’t specify how large the sample size needs to be for convergence to occur. The required sample size depends on the specific distribution of the random variables and the desired level of accuracy.
Generally, a larger sample size leads to a more accurate estimate of the expected value. This is because the impact of extreme values, or outliers, is reduced as the sample size increases. For example, if you are trying to estimate the average height of people in a population, a larger sample size will be more representative of the true average height than a smaller sample size.
Probability of Convergence
It’s important to note that the Law of Large Numbers does not guarantee a specific outcome in any given instance. Instead, it describes the probability of convergence. As the number of trials increases, the probability that the average will be close to the expected value increases, but it never reaches 100%.
For example, even with a large number of coin tosses, there is still a small probability that the observed proportion of heads will be significantly different from 50%. However, this probability decreases as the number of tosses increases.
The Law of Large Numbers is a statement about the long-run behavior of random variables, not about any particular outcome.
The Law of Large Numbers in Practice
The Law of Large Numbers (LLN) is a fundamental concept in probability and statistics, with practical applications across various fields. This section will explore how to apply the LLN in real-world scenarios, providing a step-by-step guide and illustrative examples.
Applying the Law of Large Numbers
The LLN is a powerful tool for understanding and predicting outcomes in situations involving repeated trials or observations. To apply the LLN effectively, follow these steps:
- Identify the random variable: Define the variable you are interested in measuring. This could be anything from the height of individuals in a population to the number of heads obtained in a series of coin flips.
- Determine the expected value: Calculate the expected value (or mean) of the random variable. This represents the theoretical average outcome if you were to perform an infinite number of trials.
- Collect data: Gather a large sample of data points for the random variable. The larger the sample size, the more accurate your results will be.
- Calculate the sample mean: Compute the average of the collected data points.
- Compare the sample mean to the expected value: As the sample size increases, the sample mean will converge towards the expected value. This convergence is the essence of the LLN.
Example: Using the Law of Large Numbers in Data Analysis
Imagine a company conducting a survey to understand customer satisfaction with a new product. They want to estimate the overall satisfaction level based on a sample of customer responses.
- Random variable: Customer satisfaction rating (e.g., on a scale of 1 to 5).
- Expected value: The company’s target satisfaction level, which could be based on previous product launches or industry benchmarks.
- Data collection: They collect responses from a random sample of 1000 customers.
- Sample mean: They calculate the average satisfaction rating from the sample.
- Comparison: If the sample mean is close to the expected value, the company can conclude that the overall customer satisfaction level is likely to be close to their target. As they collect data from more customers, the sample mean will become more accurate, converging towards the true population satisfaction level.
Applications in Specific Fields
Insurance
The LLN is fundamental to the insurance industry. Insurance companies use the LLN to estimate the likelihood of claims based on historical data. By analyzing a large number of past claims, they can predict the average number of claims they will receive in the future. This allows them to set premiums that are high enough to cover their expected costs while remaining competitive.
Finance
In finance, the LLN is applied in portfolio management. By diversifying investments across a wide range of assets, investors can reduce their risk. The LLN suggests that as the number of assets in a portfolio increases, the overall portfolio risk will converge towards the average risk of the individual assets. This principle is known as “diversification.”
Quality Control
The LLN is used in quality control to monitor the consistency of production processes. By taking samples from a production line and measuring key characteristics, manufacturers can estimate the overall quality of their products. If the sample data deviates significantly from the expected values, it indicates a potential problem in the production process.
Healthcare
In healthcare, the LLN is used to analyze clinical trial data. By studying a large sample of patients, researchers can estimate the effectiveness of new treatments or medications. The LLN ensures that the results of clinical trials are statistically significant, meaning that the observed effects are unlikely to be due to chance.
Ultimate Conclusion
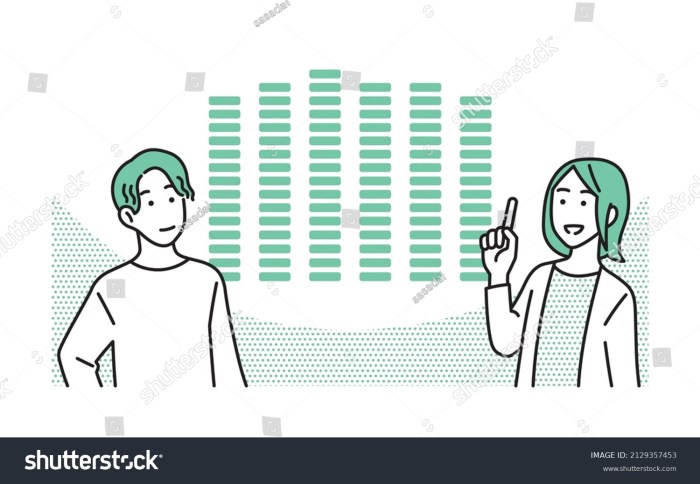
The law of large numbers provides a powerful tool for understanding and predicting the behavior of random events. It has far-reaching implications in various fields, from insurance and gambling to scientific research and data analysis. By understanding this law, we gain insights into the predictability of randomness, allowing us to make informed decisions and navigate uncertainties with greater confidence.
Questions and Answers: What Is The Law Of Large Numbers
What are some real-world examples of the law of large numbers in action?
Insurance companies use the law of large numbers to calculate premiums. By analyzing a large number of past claims, they can estimate the likelihood of future claims and set premiums accordingly. Similarly, casinos rely on the law of large numbers to ensure their profitability in the long run. The odds of winning individual games may be random, but over a large number of plays, the house edge guarantees a consistent profit for the casino.
Does the law of large numbers guarantee a specific outcome?
No, the law of large numbers does not guarantee a specific outcome. It only describes the probability of convergence. For example, while flipping a coin a thousand times will likely result in close to 500 heads, it’s still possible (though unlikely) to get a result significantly different from this.
How does the law of large numbers relate to the central limit theorem?
The central limit theorem builds upon the law of large numbers. It states that the distribution of sample means will approach a normal distribution as the sample size increases, regardless of the underlying distribution of the population. This theorem is essential for statistical inference, allowing us to draw conclusions about a population based on a sample.